In recent years many aspects of social engagement has shifted to what can be broadly thought of as online markets. These markets; which include eBay, Uber, Airbnb, Tinder, StubHub, Wikipedia, Amazon’s Mechanical Turk, etc.; are run by technology companies and are built by teams of software engineers. The science of designing and optimizing these online markets, however, is underdeveloped. Designing a marketplace that works well is challenging because the behavior of participants in the market place depends on the design of the marketplace.
Project Summaries
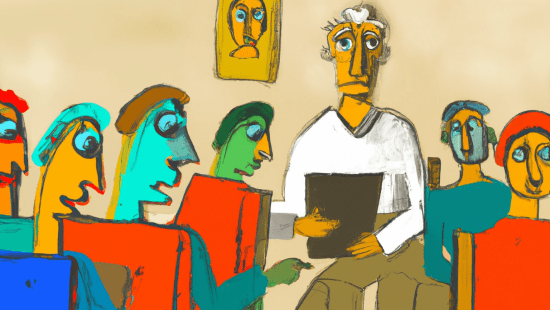
Mechanism Design for the Classroom
This project explores three main thrusts: fairness in heterogeneous grading, grading to optimize study incentives, and the design of student feedback mechanisms.
Thrust 1: Randomizing questions from a large bank of questions is a popular cheating deterrent in online exams. Thrust 2: When students are assigned tasks, their level of effort depends on how their effort is graded. Thrust 3: Feedback to students enables them to assess how their effort leads to outcomes that are relevant to them, such as learning or grades.
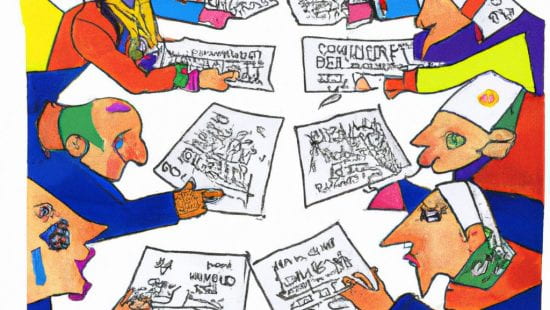
Peer Grading
A peer grading system is an online toolthat collects student submissions, and assigns review tasks to the
students and teacher, and aggregates reviews to produce assessments of
both the submissions and the peer reviews. The potential benefits of the a peer review system are learning by reviewing, reduced teacher grading, promptness of feedback, identifying early warnings. The online markets lab develops and maintains a peer grading system called PeerPal. This system is actively used in Northwestern classes.
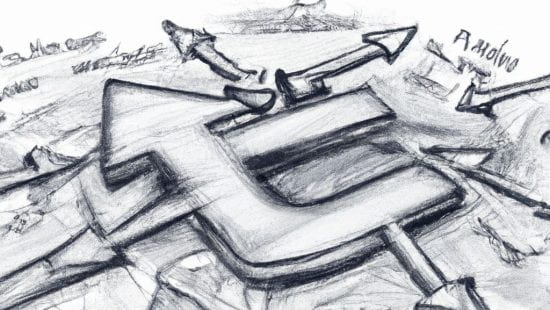
Non-truthful Mechanism Design
Online markets match participants via market mechanisms that are strategically non-transparent, a.k.a., non-truthful. There are considerably theoretical and practical challenges in designing non-truthful mechanisms that lead to good outcomes for participants. The work of the lab spans topic areas that include the design of bidding dashboards, robust analysis of outcomes in non-truthful mechanisms, and the design of good non-truthful mechanisms.
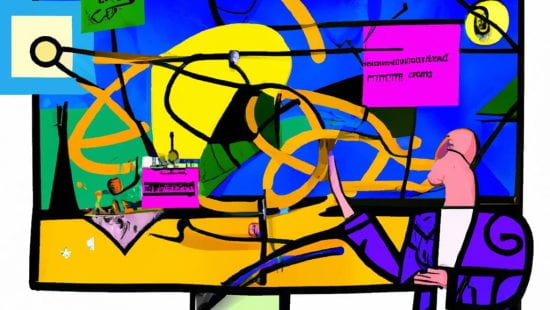
Data Science for Online Markets
Designing a marketplace that works well is challenging because the behavior of participants in the market place depends on the design of the marketplace. For example, a seller in eBay chooses among various sale formats including “buy it now” or the traditional eBay auction, how much to charge for shipping and handling, and other information to give with the listing; a buyer chooses which items to bid on, how much to bid, and when to place a bid. These actions by the participants in the marketplace are done strategically and and the choice of action depends on the propensity of the action to lead the participant to desired outcomes. Importantly, when the rules of the marketplace are changed, the actions taken by the participants may change in response. This strategic effect makes using behavioral data to understanding market outcomes and adapting the market rules — with methods from data science and machine learning — non straightforward. Data science for online markets develops methods for designing and tuning the rules of online markets from their data.